Bridging Realities: Exploring NERFS and Reality Capture Technologies in the Digital Age
In recent years, the fields of computer vision and graphics have witnessed remarkable advancements, giving rise to revolutionary technologies that have transformed various industries. Two prominent technologies that have gained significant attention are NERFS (Neural Radiance Fields) and Reality Capture. Both NERFS and Reality Capture serve as powerful tools for capturing and reconstructing the real world in a digital form. However, they differ significantly in their approaches and applications. In this article, we will delve into the main differences between NERFS and Reality Capture technologies and explore the advantages and challenges of NERF technology.
NERFS (Neural Radiance Fields):
NERFS, short for Neural Radiance Fields, is a cutting-edge technology that falls under the umbrella of computer graphics and computer vision. Developed in recent years, NERFS leverage deep learning models to represent 3D objects or scenes through a continuous 3D function. Unlike traditional methods that rely on point clouds or mesh representations, NERFS generate photo-realistic reconstructions by modeling the underlying scene as a neural network.
How NERFS Work:
NERFS work by capturing a vast number of images of a scene from different viewpoints. These images, along with the corresponding camera parameters, are fed into a neural network. The network then learns to infer the volumetric representation of the scene, encoding the intricate details, textures, and lighting conditions.
Applications of NERFS:
NERFS have shown tremendous potential in various domains, including:
Virtual Reality (VR) and Augmented Reality (AR): NERFS can create highly immersive and realistic virtual environments for VR/AR applications, enhancing user experiences.
Gaming and Entertainment: NERFS enable game developers to create realistic characters and environments, pushing the boundaries of visual fidelity in gaming.
Movie and Animation Industry: NERFS can aid in generating lifelike CGI (Computer-Generated Imagery) for movies and animations, reducing the reliance on traditional modeling and animation techniques.
Robotics and Autonomous Systems: NERFS have potential applications in robotics and autonomous systems for scene understanding and navigation.
Challenges of NERF Technology:
While NERFS hold great promise, they also come with several challenges and limitations:
Computational Complexity: NERFS require substantial computational resources for both training and inference, making them computationally intensive. Real-time applications may be limited by the complexity of the neural network.
Limited Generalization: NERFS excel at capturing scenes similar to those in their training data. However, they may struggle when presented with scenes that deviate significantly from their training data, leading to limited generalization abilities.
Memory and Storage: The volumetric nature of NERFS can lead to significant memory and storage requirements. Storing the 3D function and rendering high-resolution images can be memory-intensive.
Lack of Dynamic Scenes: NERFS are more suited for static scenes or objects due to the nature of their training data. Capturing dynamic scenes or moving objects presents a challenge as the neural network may not handle temporal changes effectively.
Conclusion:
NERFS and Reality Capture technologies are both remarkable advancements in the fields of computer graphics and vision. While NERFS focus on generating photo-realistic 3D reconstructions using neural networks, they do face challenges related to computational complexity, generalization, memory, and handling dynamic scenes. Nonetheless, their potential applications in various industries hold the promise of revolutionizing the way we interact with and perceive the digital and real worlds.
As researchers continue to address these challenges, we can expect NERF technology to evolve further, overcoming limitations and becoming even more versatile and applicable in a broader range of domains. Embracing these technological marvels and understanding their capabilities and limitations will pave the way for exciting innovations and applications that bridge the gap between reality and the digital realm.
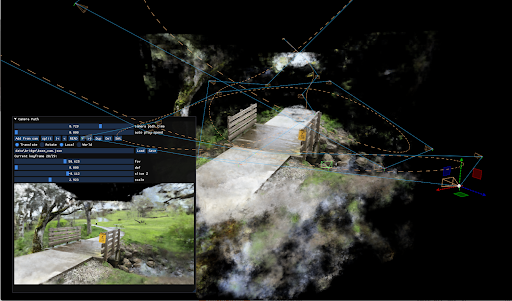
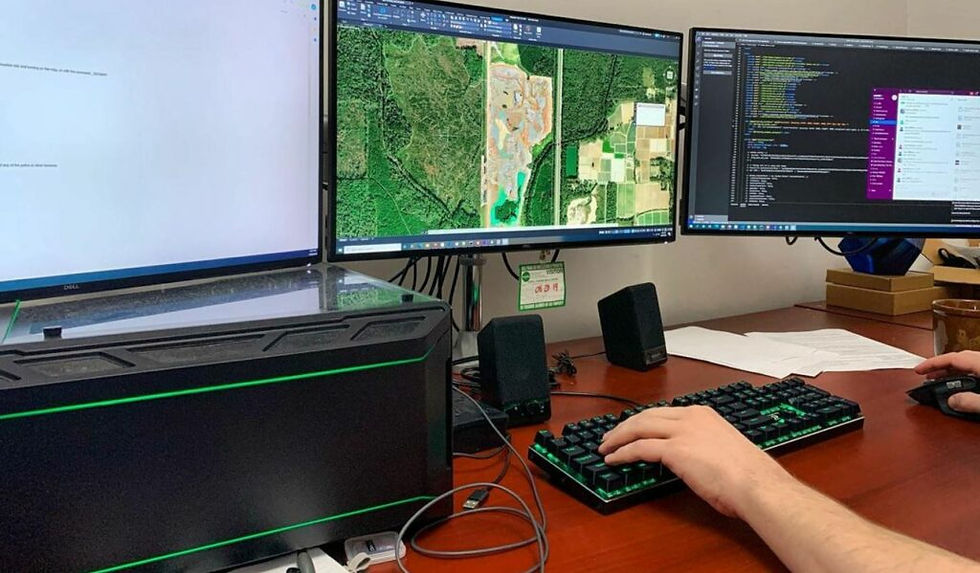
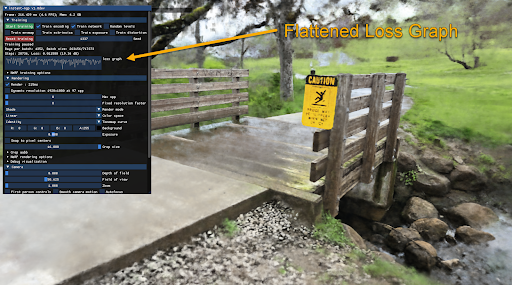
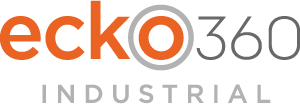
Comments